In the dynamic field of cybersecurity, where threats are becoming more complex and large-scale, integrating advanced technologies becomes essential. Cybersecurity analytics is one of these technologies that stands out for its proactive defence and insightful role. This article explores the use of data analytics tools in cybersecurity and how they enable organizations to identify anomalies, analyze patterns, and strengthen defences against a variety of cyberthreats.
Understanding Cybersecurity Analytics
Defining Cybersecurity Analytics
Cybersecurity Analytics involves the systematic analysis of vast datasets related to network activities, system logs, and user behaviors to uncover patterns, anomalies, and potential security threats. By leveraging advanced algorithms and machine learning, cybersecurity professionals gain actionable insights, allowing them to preemptively identify and mitigate cyber risks.
The Role of Data Analytics in Cybersecurity
In the context of cybersecurity, the sheer volume and complexity of data generated daily can overwhelm traditional security measures. Data Analytics Tools are designed to sift through this massive dataset, extracting meaningful information to enhance threat detection, incident response, and overall security posture.
Enhancing Threat Detection and Incident Response
1. Behavioral Analytics for Anomaly Detection
Cybersecurity Analytics employs behavioral analytics to establish baseline patterns of normal behavior within an organization’s network. Deviations from these patterns can signal potential security threats. This proactive approach enables the identification of anomalies, such as unusual access patterns or irregular data transfers, indicative of potential cyber attacks.
2. Machine Learning Algorithms for Predictive Analysis

The integration of machine learning algorithms into cybersecurity analytics enables predictive analysis. By analyzing historical data and learning from past incidents, these algorithms can forecast potential future threats. This predictive capability empowers organizations to take preemptive measures and strengthen their defenses before a cyber attack occurs.
3. Real-Time Monitoring and Threat Intelligence
Data Analytics Tools facilitate real-time monitoring of network activities, allowing cybersecurity professionals to respond promptly to emerging threats. Additionally, these tools can integrate threat intelligence feeds, providing up-to-date information about known cyber threats and enabling organizations to proactively defend against evolving attack vectors.
Data Analytics Tools in Network Security
1. Network Traffic Analysis
Data Analytics Tools analyze network traffic patterns to identify abnormalities and potential security threats. By examining the volume, frequency, and characteristics of data flows, cybersecurity professionals can pinpoint suspicious activities such as unauthorized access attempts or data exfiltration.
2. Log Analysis for Security Insights
Analyzing logs generated by various network components and systems is a fundamental aspect of cybersecurity analytics. These logs capture valuable information about user activities, system events, and potential security incidents. Data Analytics Tools can sift through these logs, providing security teams with insights into potential vulnerabilities, unauthorized access, or malicious activities.
3. User Behavior Analytics (UBA)

Understanding normal user behavior is essential for detecting anomalous activities that may indicate a security threat. User Behavior Analytics, a subset of cybersecurity analytics, utilizes data analytics tools to establish baselines for individual user activities. Deviations from these baselines can signify compromised accounts or insider threats, prompting swift investigation and response.
Endpoint Security and Data Analytics:
1. Endpoint Detection and Response (EDR)
Endpoint Security focuses on protecting individual devices connected to a network. Data Analytics Tools, integrated into Endpoint Detection and Response (EDR) solutions, analyze endpoint data to identify and respond to potential security incidents. This proactive approach enhances an organization’s ability to detect and contain threats at the device level.
2. Security Information and Event Management (SIEM)
SIEM platforms aggregate and analyze data from various sources, including endpoints, to provide a holistic view of an organization’s security landscape. By employing Data Analytics Tools, SIEM enhances threat detection by correlating events across the network, identifying patterns that may indicate a coordinated cyber attack.
Challenges and Considerations in Cybersecurity Analytics
1. Data Quality and Integration Challenges
Effectively harnessing cybersecurity analytics relies on the quality and integration of data from diverse sources. Incomplete or inconsistent data can hinder the accuracy of analytics results. Organizations must prioritize data quality and ensure seamless integration of data sources for comprehensive analysis.
2. Privacy and Ethical Considerations
As cybersecurity analytics involves analyzing vast amounts of data, organizations must navigate privacy and ethical considerations. Balancing the need for comprehensive threat detection with respect for user privacy is crucial. Implementing robust data anonymization and ensuring compliance with privacy regulations are essential components of responsible cybersecurity analytics practices.
The Future of Cybersecurity Analytics
1. Artificial Intelligence (AI) and Autonomous Security Systems

The future of cybersecurity analytics lies in the integration of artificial intelligence (AI) and the development of autonomous security systems. AI-driven analytics can enhance the speed and accuracy of threat detection, enabling autonomous systems to respond to cyber threats in real time without human intervention.
2. Quantum Computing and Cryptographic Challenges
While quantum computing holds the promise of revolutionary advancements, it also poses challenges for traditional cryptographic methods. Cybersecurity analytics will need to evolve to address the potential vulnerabilities introduced by quantum computing, necessitating the development of quantum-resistant encryption algorithms.
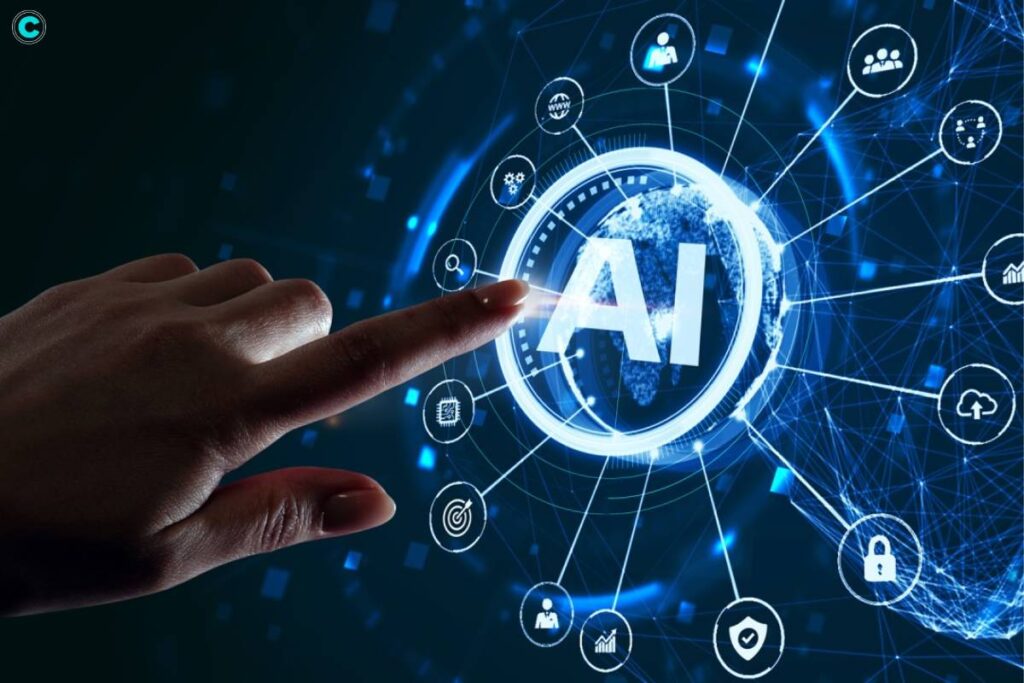
The Power of AI in Cybersecurity: A Comprehensive Analysis of Cyber Threats
Artificial intelligence (AI) improves proactive defence against cyber threats by analyzing large datasets and identifying patterns.
Conclusion: Empowering Cybersecurity Defenses with Analytics
The incorporation of Data Analytics Tools into cybersecurity operations is a game-changer in the ongoing fight against cyber attacks. Organisations are able to proactively and dynamically defend themselves against the constantly changing cyber landscape by leveraging their capacity to analyse large databases, identify abnormalities, and forecast possible threats. Organizations must adopt these tools as cybersecurity analytics develops, overcoming obstacles and moral dilemmas to strengthen their digital defences and protect sensitive data in this era of constant cyberattacks.