Imagine a world where computers can think, learn, and adapt just like the human brain. This is not a futuristic dream but a rapidly approaching reality, thanks to neuromorphic computing. As the boundaries of artificial intelligence (AI) and machine learning continue to expand, it emerges as a groundbreaking approach that promises to revolutionize how we process information. By mimicking the neural architecture of the human brain, this innovative technology aims to create more efficient, adaptive, and intelligent systems. In this article, we will explore the fascinating world of neuromorphic computing, uncovering its principles, applications, and the profound impact it is set to have on various industries.
What is Neuromorphic Computing?
Definition and Overview
It is an innovative approach to designing computer systems that mimic the human brain’s architecture and functioning. Unlike traditional computing systems that rely on binary logic and von Neumann architecture, it uses artificial neurons and synapses to process information more organically and efficiently.
History and Development
The concept of neuromorphic computing dates back to the 1980s when Carver Mead first introduced it. Over the years, significant advancements in neuroscience and materials science have propelled the development of neuromorphic systems, bringing us closer to creating machines that think and learn like humans.
The Science Behind Neuromorphic Computing
1. Biological Inspiration
It draws heavy inspiration from the structure and functioning of the human brain. The brain’s neural networks, consisting of neurons and synapses, process information in parallel, allowing for remarkable efficiency and adaptability.
2. Key Principles and Concepts
Key principles include the use of spiking neural networks (SNNs), which emulate the brain’s way of transmitting information through electrical spikes. This method not only enhances processing speed but also significantly reduces power consumption.
Neuromorphic Hardware
1. Neuromorphic Chips
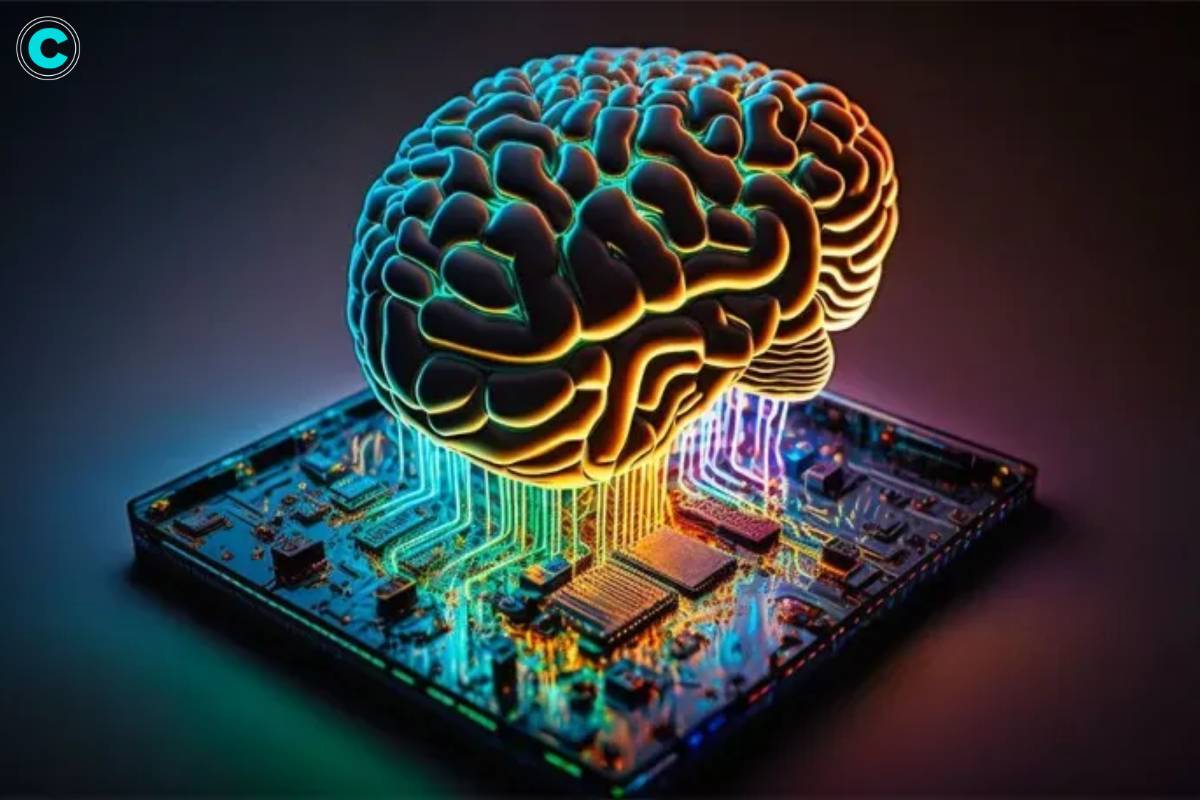
At the heart of this computing are neuromorphic chips. These specialized processors are designed to replicate the brain’s neural networks, enabling efficient and real-time data processing. Leading examples include IBM’s TrueNorth and Intel’s Loihi chips.
2. Spiking Neural Networks (SNNs)
SNNs are a crucial component of this computing. Unlike traditional neural networks, SNNs use spikes or bursts of electrical activity to transmit information. This approach closely mirrors how biological neurons communicate, leading to more efficient and realistic processing.
Advantages
1 . Energy Efficiency
One of the most significant advantages is its energy efficiency. By mimicking the brain’s low-power consumption mechanisms, neuromorphic systems can operate with significantly less energy than conventional computers.
2. Real-time Processing
Neuromorphic systems excel in real-time data processing, making them ideal for applications that require immediate responses, such as robotics and autonomous vehicles.
Applications
1. Robotics
It has the potential to revolutionize robotics by enabling machines to process sensory information and make decisions more like humans. This can lead to more adaptive and intelligent robots capable of performing complex tasks.
2. Healthcare
In healthcare, neuromorphic systems can enhance medical imaging, diagnostics, and personalized treatment plans. Their ability to process vast amounts of data in real-time can lead to more accurate and timely medical interventions.
3. Autonomous Vehicles
For autonomous vehicles, it offer faster and more efficient data processing, improving decision-making and safety. These systems can handle complex sensory inputs, such as visual and auditory data, more effectively than traditional processors.
Internet of Things (IoT)
The integration of this computing in IoT devices can lead to smarter and more responsive environments. From smart homes to industrial automation, the possibilities are endless with neuromorphic-enhanced IoT systems.
Challenges
1. Technical Hurdles
Despite its potential, it faces several technical challenges. These include the complexity of designing neuromorphic chips and the need for new programming paradigms to leverage their capabilities fully.
2. Adoption Barriers
Adoption barriers also exist, such as the lack of standardization and the need for specialized knowledge to develop and implement neuromorphic systems. Overcoming these barriers will be crucial for widespread adoption.
Comparison with Traditional Computing
1. Speed and Efficiency
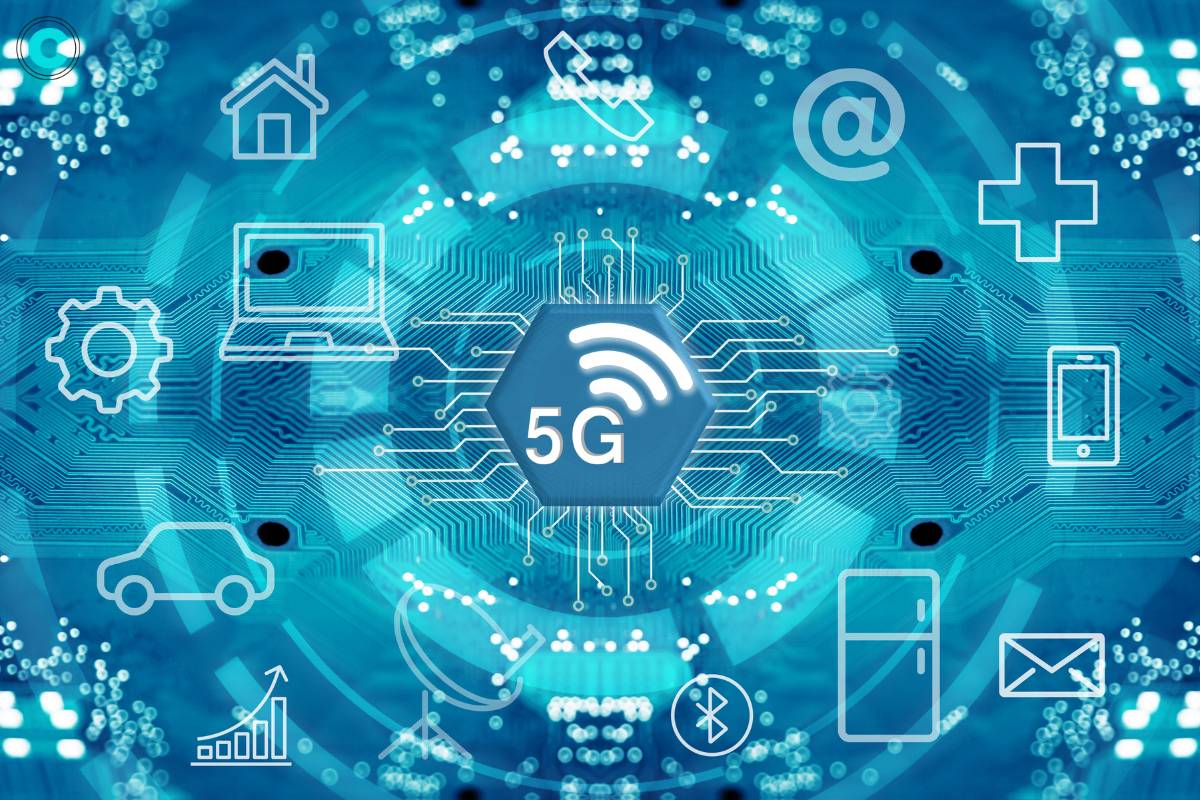
Neuromorphic systems offer superior speed and efficiency compared to traditional computers. Their parallel processing capabilities and low-power consumption make them ideal for tasks that require real-time responses.
2. Scalability
Scalability is another area where neuromorphic computing shines. Unlike traditional systems that struggle with scaling, neuromorphic architectures can easily expand to accommodate larger and more complex tasks.
Future Prospects of Neuromorphic Computing
1. Research and Development
Ongoing research and development in this computing are paving the way for even more advanced and efficient systems. Collaboration between neuroscientists, computer scientists, and engineers is driving innovation in this field.
2. Potential Impact on AI
The potential impact of this computing on AI is immense. By creating systems that learn and adapt like the human brain, we can develop more intelligent and capable AI applications that can solve complex problems in ways that traditional AI cannot.
Leading Companies
1. IBM
IBM is a pioneer in neuromorphic computing, with its TrueNorth chip leading the way. This chip features millions of artificial neurons and synapses, enabling advanced cognitive computing capabilities.
2. Intel
Intel’s Loihi chip is another significant player in the neuromorphic space. Loihi is designed to emulate the brain’s natural learning processes, making it ideal for applications that require adaptive and intelligent systems.
3. BrainChip Holdings
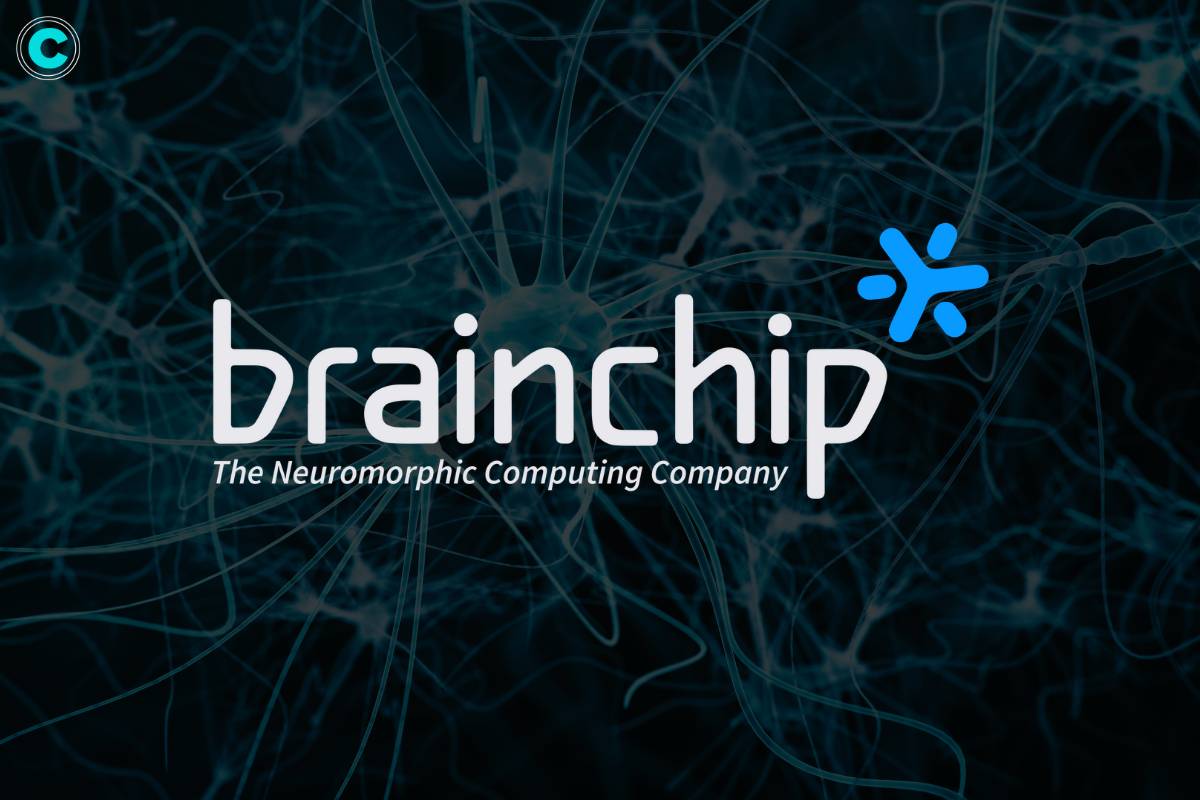
BrainChip Holdings is known for its Akida neuromorphic system, which offers real-time learning and inference capabilities. Akida is designed for edge AI applications, providing efficient and low-power solutions for various industries.
Case Studies and Real-world Examples
Case studies and real-world examples highlight the practical applications of this computing. From improving medical diagnostics to enhancing autonomous vehicle navigation, the impact of neuromorphic systems is being felt across various sectors.
Neuromorphic Computing and Ethics
1. Privacy Concerns
As with any advanced technology, it raises privacy concerns. The ability to process and analyze vast amounts of data in real-time necessitates robust privacy protections to ensure user data is safeguarded.
2. Ethical Implications
Ethical implications also arise from the use of neuromorphic systems. Questions about the potential for misuse and the need for ethical guidelines to govern their development and deployment are critical considerations.
Neuromorphic Computing in Education
1. Training and Development Programs
To foster growth, education, and training programs are essential. Universities and institutions are beginning to offer specialized courses and programs to equip the next generation of scientists and engineers with the skills needed to advance this field.
How to Get Started?
Resources and Learning Paths
For those interested in this computing, various resources and learning paths are available. Online courses, workshops, and research papers provide valuable insights and knowledge to help you get started in this exciting field.
FAQs
1. What is neuromorphic computing?
It is a field of computing that aims to mimic the neural architecture and functioning of the human brain to create more efficient and intelligent systems.
2. How does it differ from traditional computing?
Unlike traditional computing, which relies on binary logic and von Neumann architecture, it uses artificial neurons and synapses to process information in a way that closely resembles the human brain.
3. What are the advantages of neuromorphic computing?
It offers several advantages, including energy efficiency, real-time processing, and scalability, making it ideal for applications that require immediate and adaptive responses.
4. Which industries can benefit from neuromorphic computing?
Industries such as healthcare, robotics, autonomous vehicles, and the Internet of Things (IoT) can benefit significantly from the advancements in neuromorphic computing.
5. What are the challenges facing neuromorphic computing?
Challenges include technical hurdles in designing neuromorphic chips, adoption barriers, and the need for new programming paradigms to fully leverage the capabilities of
neuromorphic systems.
Conclusion
Neuromorphic computing represents a groundbreaking shift in how we approach computing and AI. By emulating the human brain’s architecture and functioning, neuromorphic systems offer unparalleled efficiency, real-time processing, and adaptability. As research and development continue to advance, the potential applications of this computing are vast, promising to revolutionize industries ranging from healthcare to autonomous vehicles. Embracing this technology and overcoming its challenges will pave the way for a smarter, more efficient future.