In the rapidly evolving landscape of machine learning, staying ahead of the curve often means leveraging innovative approaches to optimize model performance and efficiency. Active machine learning stands out as one such approach, offering a dynamic framework for selecting and labeling the most informative data points to drive learning and decision-making processes. In this article, we’ll delve deeper into the concept of active machine learning, examining its underlying principles, practical applications, benefits, challenges, and emerging trends shaping its future.
Understanding Active Machine Learning:
At its core, active machine learning is a paradigm where algorithms proactively select the most informative instances from an unlabeled dataset to query for labels. Unlike traditional passive learning approaches that rely on pre-labeled data, active learning algorithms actively engage in the labeling process, strategically choosing samples that are expected to maximize learning efficiency.
Key Components of Active Machine Learning:

1. Query Strategy:
The heart of active learning lies in the selection of data points for labeling, a process governed by various query strategies. These strategies, such as uncertainty sampling, query-by-committee, and stream-based selection, aim to identify instances that offer the greatest learning value, often based on measures of uncertainty, diversity, or model disagreement.
2. Model Training:
Active learning unfolds through an iterative cycle of data selection, labeling, and model refinement. Starting with a small labeled dataset, the algorithm incrementally enhances its performance by incorporating newly labeled instances, updating the model parameters, and iteratively refining its predictive capabilities.
3. Human-in-the-Loop:
While active learning algorithms excel at selecting informative samples, human expertise remains invaluable in guiding the labeling process. Human-in-the-loop frameworks empower domain experts to provide labels for selected instances, ensuring the algorithm focuses on acquiring high-quality annotations that align with specific application requirements.
Applications of Active Machine Learning:

1. Text Classification and Sentiment Analysis
In the realm of natural language processing, active machine learning facilitates efficient text classification and sentiment analysis by prioritizing the labeling of documents or snippets that offer the greatest insights into underlying patterns and sentiments.
2. Image Annotation and Object Detection
Active learning plays a crucial role in image annotation tasks, where algorithms intelligently select images or regions of interest for annotation, enhancing the training of image recognition and object detection models with minimal manual effort.
3. Anomaly Detection and Fraud Prevention
In security-sensitive domains such as finance and cybersecurity, active machine learning aids in anomaly detection and fraud prevention by identifying suspicious or atypical patterns in data, enabling proactive intervention and risk mitigation.
4. Healthcare and Medical Imaging
Active learning holds promise in healthcare applications, particularly in medical imaging analysis, where it helps prioritize the annotation of clinically relevant images, assisting healthcare professionals in diagnosis, treatment planning, and disease monitoring.
5. Recommendation Systems and Personalization

Active learning techniques are instrumental in recommendation systems, where they optimize user engagement and satisfaction by selecting personalized recommendations tailored to individual preferences, behaviors, and feedback.
FAQs (Frequently Asked Questions)
1. What distinguishes active machine learning from traditional machine learning approaches?
It differs from traditional approaches by actively selecting data points for labeling, allowing algorithms to prioritize the acquisition of high-information instances and optimize learning efficiency.
2. How does active machine learning benefit from human expertise?
Human-in-the-loop frameworks complement active learning by leveraging domain experts to provide high-quality labels for selected instances, ensuring that the algorithm focuses on acquiring annotations that align with specific application requirements.
3. What factors influence the choice of query strategy in active machine learning?
Query strategies in active learning vary based on factors such as the nature of the data, the learning task, and the available computational resources, with strategies like uncertainty sampling, query-by-committee, and diversity sampling offering distinct trade-offs in terms of effectiveness and efficiency.
4. What are the key challenges associated with active machine learning?
Challenges in active machine learning include the design of effective query strategies, the management of human annotation efforts, the handling of noisy or unreliable labels, and the scalability of algorithms to large-scale datasets and real-world applications.
5. How does active machine learning contribute to model performance and efficiency?
It enhances model performance and efficiency by optimizing the selection of informative data points for labeling, enabling algorithms to learn from fewer labeled instances, reduce manual annotation effort, and achieve higher accuracy with minimal supervision.
Conclusion:
In summary, active machine learning emerges as a transformative paradigm in the realm of machine learning, offering multifaceted benefits that resonate across a wide spectrum of industries and use cases. By strategically identifying and labeling data points, active learning algorithms empower organizations to streamline the learning process, enhance model accuracy, and mitigate the laborious burden of manual annotation. Furthermore, the synergy between machine intelligence and human expertise fosters a collaborative ecosystem where insights are gleaned and refined through iterative feedback loops.
Its versatility extends beyond traditional boundaries, permeating diverse domains ranging from healthcare and finance to cybersecurity and beyond. Its adaptive nature enables dynamic responses to evolving data landscapes, equipping decision-makers with actionable insights and foresight into emerging trends and patterns. As active machine learning continues to evolve and assimilate with cutting-edge technologies such as deep learning and reinforcement learning, its potential for innovation and disruption knows no bounds.
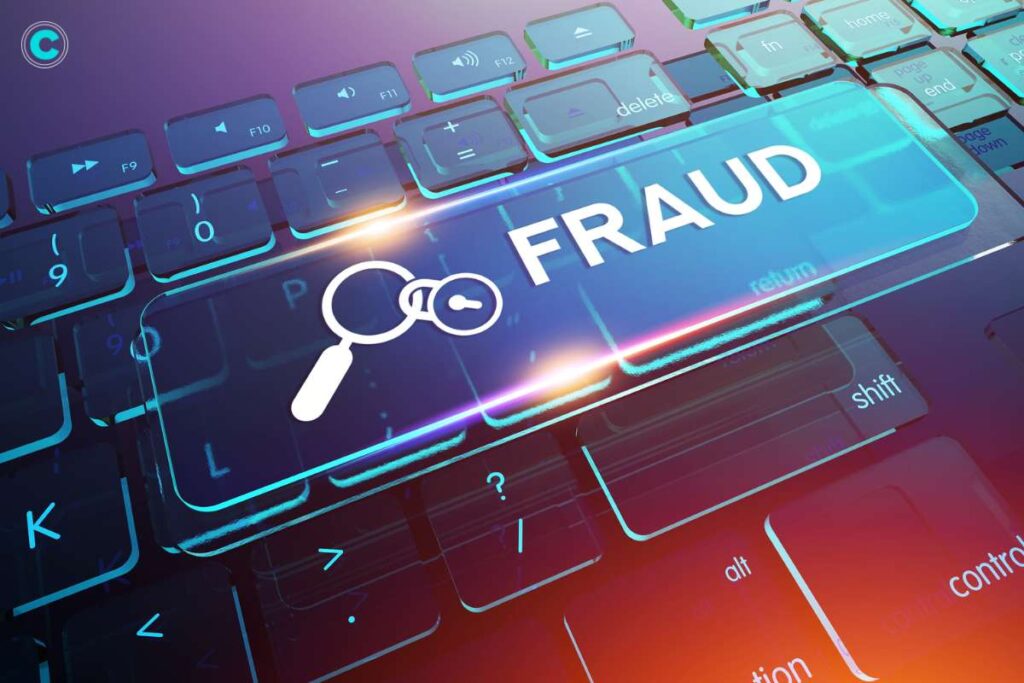
Boosting Security: Fraud Detection Using Machine Learning
In the digital age, where financial transactions are conducted online at an unprecedented scale, the risk of fraudulent activities looms large. From credit card fraud and
Moreover, the democratization of active learning frameworks paves the way for broader adoption and accessibility, democratizing access to advanced analytical capabilities and fostering a culture of innovation and experimentation. By democratizing access to advanced analytical capabilities and fostering a culture of innovation and experimentation, active machine learning becomes a catalyst for progress and transformation, fueling breakthroughs in research, industry, and society at large.
In essence, active machine learning stands poised at the vanguard of technological innovation, heralding a new era of intelligent decision-making, and unlocking unprecedented opportunities for growth and advancement. As we navigate the complex landscape of data-driven decision-making, active learning serves as a beacon of promise, illuminating the path toward a future where human ingenuity and machine intelligence converge to shape a better tomorrow.