In today’s digital age, where online communication reigns supreme, understanding human sentiments expressed in textual data has become a critical task for businesses, researchers, and analysts alike. Sentiment analysis, also known as opinion mining, leverages the power of natural language processing (NLP) and machine learning to extract insights from text, uncovering the emotions, opinions, and attitudes of individuals. With the advent of deep learning, sentiment analysis has undergone a significant evolution, revolutionizing the way we analyze and interpret textual data. In this comprehensive guide, we delve into the intricacies of sentiment analysis using deep learning, exploring its methodologies, applications, and future implications.
Unraveling Sentiment Analysis Using Deep Learning: A Comprehensive Overview:

Sentiment analysis involves the process of classifying textual data into predefined categories, such as positive, negative, or neutral sentiments. Traditional sentiment analysis methods often relied on lexicon-based approaches or machine learning algorithms trained on handcrafted features. However, these methods struggled to capture the nuances and complexities of human language, leading to suboptimal performance in real-world scenarios.
Enter deep learning – a subset of machine learning that utilizes neural networks with multiple layers to extract high-level features from raw data. Deep learning models, particularly recurrent neural networks (RNNs) and transformer-based architectures like BERT (Bidirectional Encoder Representations from Transformers), have demonstrated remarkable success in various NLP tasks, including sentiment analysis.
Applications of Sentiment Analysis Using Deep Learning:
The applications of sentiment analysis using deep learning are vast and diverse, spanning across industries and domains:
1. Social Media Monitoring:
Social media platforms serve as rich sources of textual data, with millions of users expressing their opinions and sentiments on a wide range of topics. Deep learning models can analyze this data in real time, providing valuable insights into public opinion, trending topics, and brand sentiment.
2. Customer Feedback Analysis:
Businesses can leverage sentiment analysis to analyze customer feedback, reviews, and surveys. By understanding customer sentiment, companies can identify areas for improvement, address customer concerns, and enhance overall satisfaction.
3. Market Research and Competitive Analysis:

Deep learning-powered sentiment analysis enables market researchers to gain insights into consumer preferences, market trends, and competitor strategies. By analyzing online discussions, reviews, and social media conversations, businesses can stay ahead of the competition and make data-driven decisions.
4. Financial Market Analysis:
Sentiment analysis using deep learning has applications in financial markets, where it is used to analyze news articles, social media posts, and financial reports to gauge investor sentiment and market sentiment. This information can be valuable for making investment decisions, predicting market trends, and managing risk.
5. Healthcare and Patient Feedback:
In the healthcare industry, sentiment analysis can be used to analyze patient feedback, reviews, and medical records. By understanding patient sentiments, healthcare providers can improve patient experiences, enhance care delivery, and identify areas for improvement.
How Deep Learning Enhances Sentiment Analysis:

Deep learning models have several advantages over traditional machine learning algorithms in sentiment analysis:
1. Capturing Contextual Information:
Deep learning models, such as RNNs and transformers, are capable of capturing contextual information from text, allowing them to understand the relationships between words and phrases. This enables more accurate sentiment classification, especially in cases where context is crucial.
2. Feature Learning:
Deep learning models automatically learn high-level features from raw data, eliminating the need for manual feature engineering. This enables them to extract relevant information from text without the need for extensive preprocessing.
3. Scalability:
Deep learning models can scale to handle large volumes of data, making them suitable for analyzing massive datasets commonly found in social media, e-commerce, and other online platforms.
FAQs (Frequently Asked Questions)
1. How does deep learning improve sentiment analysis accuracy?
Deep learning models excel at capturing contextual information and nuances in language, leading to more accurate sentiment classification compared to traditional approaches.
2. Can sentiment analysis using deep learning handle multilingual content?
Yes, deep learning models can be trained on multilingual datasets, enabling them to analyze sentiment in various languages. This adaptability makes them suitable for diverse global applications.
3. What role does data preprocessing play in sentiment analysis using deep learning?
Data preprocessing is crucial for removing noise, handling outliers, and tokenizing text. Clean, well-processed data enhances the performance of deep learning models in sentiment analysis.
4. Are deep learning models resilient to sarcastic or ironic expressions in sentiment analysis?
While deep learning models can capture context and nuances, handling sarcasm or irony can still pose challenges. Training models with diverse datasets that include such expressions helps improve their robustness.
5. How can businesses leverage sentiment analysis using deep learning for competitive advantage?
Businesses can use sentiment analysis to gain insights into customer opinions, enhance products or services based on feedback, monitor brand reputation, and make data-driven decisions, ultimately gaining a competitive edge.
In Summary: The Future of Sentiment Analysis Using Deep Learning
Sentiment analysis, propelled by advancements in deep learning, has not only revolutionized the way businesses understand consumer sentiments but has also opened up new avenues for researchers and analysts to glean insights from textual data. With each leap forward in deep learning technology, sentiment analysis models are becoming increasingly adept at deciphering the intricacies of language and context, allowing for more nuanced and accurate analyses.
Looking ahead, the future of sentiment analysis using deep learning holds immense promise. As researchers continue to refine algorithms and techniques, we can anticipate the emergence of even more sophisticated models capable of discerning subtle emotional nuances and cultural context. These advancements will not only enhance the accuracy and efficiency of sentiment analysis but will also unlock deeper insights into human behavior and opinion.
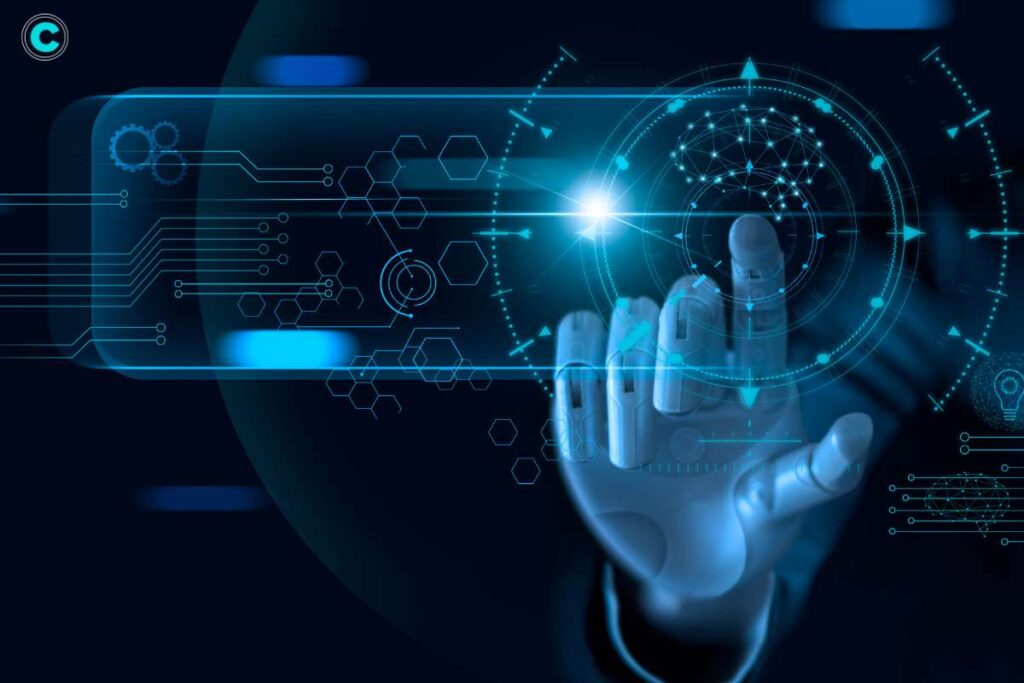
Exploring Deep Machine Learning Books: Your Ultimate Guide to Advanced AI Techniques
Deep machine learning books are authoritative resources that delve into the theory, algorithms, and applications of deep learning techniques.
Moreover, the integration of deep learning with other cutting-edge technologies such as natural language processing (NLP), computer vision, and predictive analytics will further augment the capabilities of sentiment analysis systems. This interdisciplinary approach will enable businesses to extract richer insights from a variety of data sources, including text, images, and videos, fostering a more holistic understanding of consumer sentiment and behavior.
In conclusion, the future of sentiment analysis using deep learning is bright, promising more accurate, efficient, and insightful analyses of human emotions and opinions in the digital age. As businesses and researchers continue to harness the power of deep learning, we can expect sentiment analysis to play an increasingly pivotal role in shaping decision-making processes, driving innovation, and ultimately, enhancing the overall human experience in the digital era.