(Source – Pandio)
In today’s tech-savvy world, you might have heard people talking about “AI infrastructure”. It’s a fancy term that basically means having a strong foundation for using artificial intelligence (AI) in businesses. AI is changing the way industries work, so having a solid AI infrastructure is super important for companies that want to use this technology effectively. This article will explain why it’s so important, and some tips for building a strong one. It’s all about helping organizations stay competitive and efficient in this AI-focused world we’re living in.
Understanding AI Infrastructure
It refers to the combination of hardware, software, data storage, and networking resources required to support AI applications. It encompasses everything from powerful GPUs and TPUs, to data management systems, to cloud computing services. An efficient artificial intelligence infrastructure is vital for the development, training, and deployment of AI models, ensuring they can perform complex computations and process vast amounts of data quickly and accurately.
Key Components of AI Infrastructure
1. Computing Power
- GPUs and TPUs: Graphics Processing Units (GPUs) and Tensor Processing Units (TPUs) are essential for handling the heavy computational loads of AI algorithms. They enable faster processing and training of AI models.
- CPUs: Central Processing Units (CPUs) still play a significant role in running AI workloads, particularly for less intensive tasks.
- FPGA: Field Programmable Gate Arrays (FPGAs) offer flexibility and efficiency for specific AI applications, allowing customization of hardware to optimize performance.
2. Data Storage and Management

- Databases: Efficient databases are necessary for storing and retrieving large datasets used in training AI models.
- Data Lakes: These are vast storage repositories that hold raw data in its native format until needed, providing a cost-effective solution for managing big data.
- Data Warehouses: These are optimized for querying and analyzing structured data, helping organizations make informed decisions based on AI insights.
3. Networking
- High-Speed Connectivity: Fast and reliable network connections are critical for transferring large volumes of data between different components of the artificial intelligence infrastructure.
- Edge Computing: This involves processing data closer to where it is generated, reducing latency and improving real-time decision-making capabilities.
4. Software and Frameworks
- AI Frameworks: TensorFlow, PyTorch, and other AI frameworks provide essential tools and libraries for building and training AI models.
- Middleware: Middleware solutions facilitate communication and data exchange between different parts of the artificial intelligence infrastructure, ensuring seamless integration.
5. Cloud Services
- Public Cloud: Providers like AWS, Google Cloud, and Microsoft Azure offer scalable and flexible resources for AI development.
- Private Cloud: Dedicated cloud environments provide enhanced security and control over it.
- Hybrid Cloud: Combines the benefits of both public and private clouds, offering a balanced approach to scalability, security, and cost.
Importance of Robust AI Infrastructure
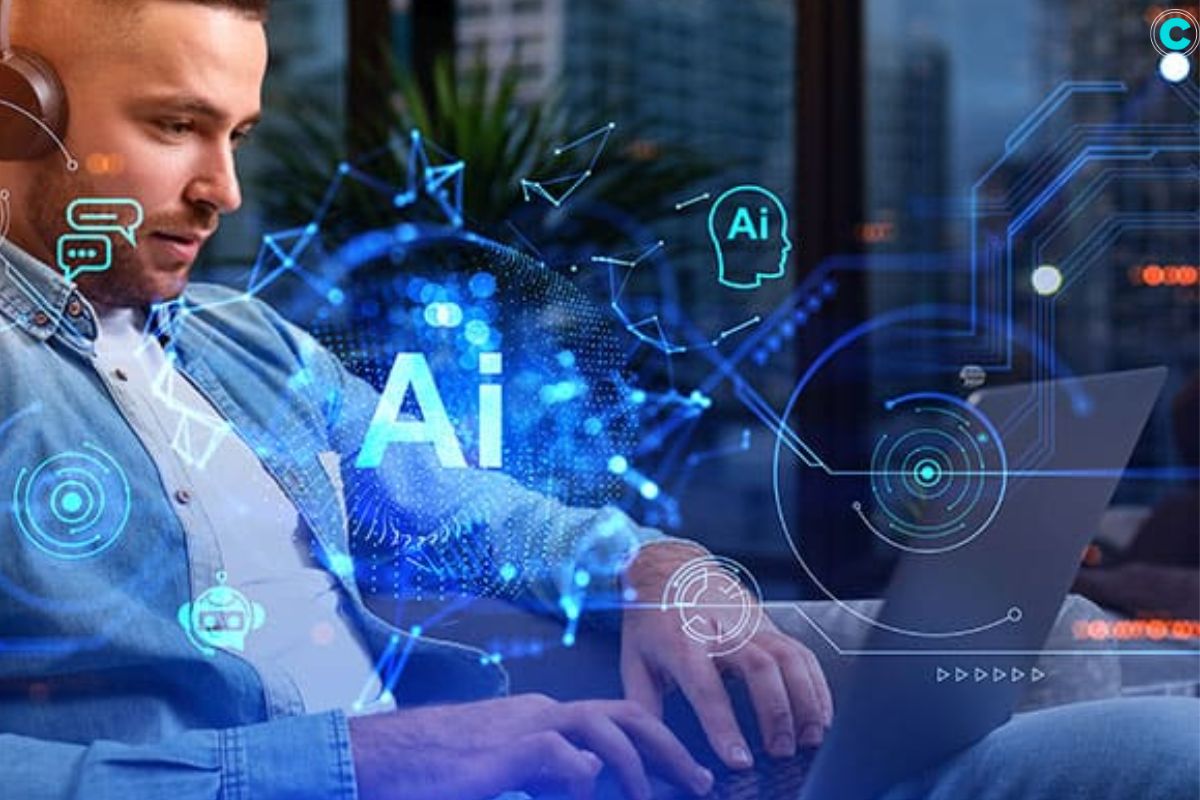
- Scalability: As AI projects grow, the infrastructure must be able to scale to accommodate increased computational demands and data volumes.
- Performance: Efficient artificial intelligence infrastructure ensures high performance, reducing the time required to train and deploy models.
- Cost-Effectiveness: Optimizing infrastructure helps in managing costs, especially when using cloud services where pay-as-you-go models are common.
- Security: Proper infrastructure setup can safeguard sensitive data and ensure compliance with regulatory standards.
- Innovation: A strong artificial intelligence infrastructure fosters innovation by providing the necessary tools and resources for developing cutting-edge AI applications.
Best Practices for Developing AI Infrastructure
1. Assess Business Needs
- Understand the specific AI requirements of your business. This includes the type of AI applications, data volume, and computational needs.
2. Invest in Scalable Solutions
- Choose infrastructure components that can grow with your business. This includes scalable cloud services, modular hardware, and flexible data management solutions.
3. Optimize Data Management
- Implement efficient data storage solutions like data lakes and warehouses to manage and utilize data effectively.
- Ensure proper data governance and security measures are in place.
4. Leverage Cloud Computing
- Utilize cloud services for flexibility and scalability. Hybrid cloud solutions can offer a balance between cost efficiency and control.
5. Focus on Security

- Incorporate robust security protocols to protect data integrity and privacy.
- Regularly update and patch infrastructure components to prevent vulnerabilities.
6. Train and Upskill Teams
- Ensure your team is skilled in using AI tools and managing artificial intelligence infrastructure. Regular training and upskilling are essential in keeping up with technological advancements.
The Future of AI Infrastructure
As AI continues to advance, the demands on artificial intelligence infrastructure will only increase. Emerging technologies like quantum computing, advanced neural networks, and more sophisticated AI models will require even more robust and flexible infrastructure solutions. Organizations must stay ahead of the curve by continuously investing in and upgrading their artificial intelligence infrastructure.
FAQs
1. What is AI infrastructure?
It refers to the combined hardware, software, data storage, and networking resources needed to develop, train, and deploy AI models effectively.
2. Why is computing power important in AI infrastructure?
Computing power, especially from GPUs and TPUs, is essential for processing the large volumes of data and complex calculations required by AI algorithms, enabling faster and more efficient model training and execution.
3. How do cloud services contribute to AI infrastructure?
Cloud services provide scalable and flexible resources that can be easily adjusted based on demand, making them ideal for AI projects with varying computational and storage needs. They offer cost-effective solutions and ease of access to advanced tools and frameworks.
4. What role does data management play in AI infrastructure?
Effective data management ensures that large datasets are stored, organized, and accessed efficiently. This is crucial for training AI models and deriving meaningful insights, as well as maintaining data security and compliance.
5. How can businesses ensure the security of their AI infrastructure?
Businesses can ensure security by implementing robust protocols, regularly updating and patching systems, encrypting sensitive data, and following best practices for data governance. Employing dedicated security measures and monitoring can further protect artificial intelligence infrastructure from threats.
Conclusion
By focusing on these key aspects, businesses can build and maintain a strong AI infrastructure that supports their AI initiatives, driving innovation and competitive advantage in their respective industries.